Physical activity (PA) is one of the lifestyle factors that most influence whether people live a long and healthy life. Not only does an active lifestyle make people live longer,but it also contributes to better mental health and, in general, a higher quality of life.
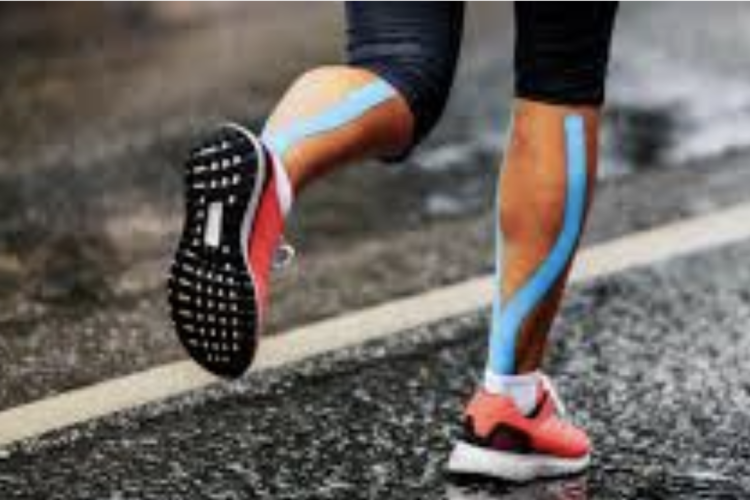
Author: Alessio Rossi (UniPi)
Warm-up (WU) represents one of the most employed pre-exercise routines anticipating a physical activity, both in health-related and competitive sporting environments. It is widely considered effective to promote specific effects related (e.g., increased thermoregulatory strain and decreased resistance of muscles and joints) and non-related (e.g., post-activation potentiation and psychological effects) to temperature changes.
"I would like us to move towards an Artificial Intelligence centered on the person and society. [...] The choices we face today are linked to fundamental ethical issues about the impact of AI on society, in particular its influence on work, social interactions, healthcare, privacy and equity."
Interesting interview with Fosca Giannotti on the future of AI and SoBigData.
https://www.donnenellascienza.it/news/interviste/fosca-giannotti/
In many data science tasks our goal is to understand the relationship of two different sets of entities. For example, a common problem that online shops need to solve is to understand the relationship between customers and products. This relationship reveals clusters of products that are frequently bought together and groups of users who buy similar products. Such insights can then be used for marketing activities or to make product recommendations.
Online social networks are an integral part of modern society: they connect millions of users from around the world. For many people they serve as their main news aggregators and a window of what is happening in the world. Despite intensive research, many phenomena revolving around online social networks and their impact on society are still not sufficiently understood. One of the main challenges in this area is to establish whether echo chambers are widely present in social networks or not.
The estimation of air pollutants’ distribution over space is a significant challenge that concerns climate change and human health. Greenhouse gas (GHG) emissions from the transport sector have more than doubled since 1970, and about 80% of this growth has come from road transport [1]. In 2016, 11.9% of global GHG emissions were from road transport (60% of which from passenger travel and 40% from road freight) [2].
The new national PhD program in Artificial Intelligence is on the launchpad!
Our KDD Lab - Knowledge Discovery & Data Mining Laboratory @ University of Pisa and ISTI-CNR of the National Research Council of Italy funds 3 positions at the "AI & Society" PhD.
Interested to join a vibrant research ecosystem on cutting edge topics around on human centered AI, XAI, social AI, AI ethics and Trustworthy AI?
Our cities stand today as a miraculous lab to foster an effective joint effort of different disciplines united by the common goal of setting our cities on a path towards well-being and social and environmental sustainability.